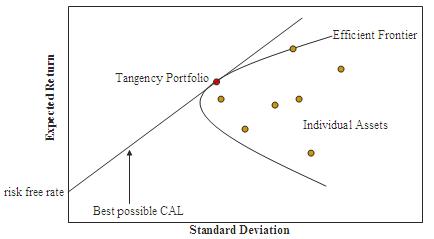
The Problem with Modern Portfolio Theory
The Problem with Modern Portfolio Theory
The world of finance is replete with theories and models. Yet, few concepts have left as indelible a mark as Modern Portfolio Theory (MPT).
Proposed by Harry Markowitz in his 1952 paper, “Portfolio Selection,” MPT has been both a guiding light and a point of contention among investors for decades.
What is Modern Portfolio Theory?
An example of the relationship between different asset classes
Modern Portfolio Theory revolves around the idea of diversification — essentially, the age-old wisdom of not putting all your eggs in one basket.
At its core, MPT posits that the risk and return of an overall portfolio are more important than the risk and return of individual assets.
Foundational Concepts:
- Expected Returns: An anticipated value for the return on an investment, often calculated based on historical data.
- Portfolio Volatility: The standard deviation of portfolio returns, representing the total risk of the portfolio.
- Efficient Frontier: A curve that defines the portfolios offering the highest expected return for a given level of risk (or the lowest risk for a given level of return).
The primary tenet is that a diversified portfolio can be constructed to optimize returns for a given level of risk or, conversely, minimize risks for a desired return. This optimization process leads to the efficient frontier, where portfolios lie on a curve representing the best risk-return trade-offs.
How Does Modern Portfolio Theory Work?
source: Wikipedia
Imagine you have multiple assets to invest in, each with its respective return and risk profile. According to MPT:
- Diversification Benefits: By diversifying across assets that are not perfectly correlated, you can reduce the portfolio’s overall risk without necessarily sacrificing returns. This happens because individual asset volatilities can offset each other.
- Optimal Portfolio Creation: For a given risk level, there exists an optimal combination of assets that will offer the highest possible return. This combination forms the efficient frontier.
- Risk-Free Assets & Capital Allocation Line: Introducing a risk-free asset (like a treasury bill) allows for a combination of the risk-free asset and a portfolio on the efficient frontier, leading to a straight line known as the capital allocation line. The point where this line is tangent to the efficient frontier is the market portfolio.
This approach emphasizes the collective behavior of assets, acknowledging that individual asset behavior isn’t as crucial when viewed within the context of an entire portfolio.
The Problem with Modern Portfolio Theory
While Modern Portfolio Theory (MPT) has been a pillar of finance for decades, as financial markets have evolved and academic research has delved deeper into investor behavior and market dynamics, various criticisms have emerged.
Asset correlations can rapidly change during a bear market
1. Assumption of Rationality:
- Description: Central to MPT is the assumption that investors are rational actors who aim to maximize their utility (usually represented by expected returns) for a given level of risk.
- Reality and Examples: Behavioral finance has identified numerous instances where investors deviate from rationality. For example, during the Japanese asset price bubble in the late 1980s, investors continued to buy into skyrocketing real estate and stock prices, even when fundamental valuations could not justify such prices1.
- Academic Insight: Kahneman and Tversky’s Prospect Theory illustrates how people make decisions involving probabilities. They found that investors often overvalue potential losses compared to potential gains, leading to irrational decision-making2.
2. Dependence on Historical Data:
- Description: MPT models rely heavily on historical data to estimate expected returns, variances, and covariances.
- Reality and Examples: Markets, economies, and geopolitical scenarios evolve. The historical data from emerging markets, such as Brazil’s stock market in the 1990s, may not capture the entire range of potential future outcomes, given the rapidly changing economic environment and institutional reforms during that period3.
- Academic Insight: Ibbotson and Kaplan, in their 2000 study, discussed how historical returns are often poor predictors of future returns due to changing market conditions4.
3. Static Correlations:
- Description: MPT assumes that correlations between assets remain consistent.
- Reality and Examples: The 1997 Asian Financial Crisis saw previously uncorrelated economies and markets fall in tandem. For instance, while South Korea and Thailand had different economic structures, both faced massive capital outflows and devaluations, demonstrating converging correlations during crises5.
- Academic Insight: Longin and Solnik’s study on international equity markets showed that correlations between markets increase in volatile conditions, challenging MPT’s static correlation assumption6.
4. Over-reliance on Quantitative Analysis:
- Description: MPT is rooted in quantitative data, potentially sidelining qualitative factors.
- Reality and Examples: The downfall of Long-Term Capital Management (LTCM) in 1998 is a case in point. Despite the hedge fund being run by two Nobel Prize-winning economists and employing sophisticated models, they overlooked political and operational risks during the Russian financial crisis7.
- Academic Insight: Daniel and Titman’s 1997 study illustrated that stock returns were more closely linked to firm characteristics than to their beta coefficients, emphasizing the importance of qualitative factors8.
5. Over-Simplification of Investor Goals and Constraints:
- Description: MPT is rooted in the idea that risk and return are the primary considerations for investors.
- Reality and Examples: In the late 2000s, many pension funds across Europe shifted to more conservative assets, not just due to risk-return trade-offs, but due to regulatory pressures, liquidity needs, and long-term liabilities9.
- Academic Insight: A study by Ang, Papanikolaou, and Westerfield highlighted how investor objectives and constraints, such as labor income risks, can influence portfolio decisions beyond mere risk-return considerations10.
MPT Failures in the US Stock Market
1. The 1987 Stock Market Crash (Black Monday):
- What Happened: On October 19, 1987, U.S. stock markets witnessed their most significant one-day percentage drop in history, with the Dow Jones Industrial Average plummeting by 22.6%.
- MPT Shortcoming: MPT assumes a normal distribution of asset returns, but Black Monday defied this assumption, representing a multi-standard deviation event that was considered nearly impossible based on traditional models.
2. The Tech Bubble Burst (2000-2002):
- What Happened: At the turn of the millennium, the dot-com bubble, characterized by exuberantly valued tech stocks, burst. Between 2000 and 2002, the NASDAQ Composite, which had many of these tech stocks, lost 78% of its value.
- MPT Shortcoming: The bursting of the bubble showed that diversifying across sectors isn’t always sufficient. Many investors, believing they were adequately diversified, still faced substantial losses because various sectors were indirectly influenced by the tech sector’s downturn.
3. The 2008 Financial Crisis:
- What Happened: Triggered by the collapse of large financial institutions due to exposure to subprime mortgages, it resulted in sharp declines in consumer wealth, severe disruptions in financial markets, and the onset of a deep recession.
- MPT Shortcoming: Asset correlations, which are central to MPT, converged during the crisis. Diversification benefits diminished as a wide variety of assets, from stocks to real estate, all fell in tandem, challenging MPT’s foundational premise.
4. Long-Term Capital Management (LTCM) Crisis (1998):
- What Happened: LTCM, a hedge fund managed by two Nobel Prize-winning economists who heavily relied on advanced financial models, faced catastrophic losses during the Russian financial crisis.
- MPT Shortcoming: Despite the use of sophisticated models rooted in MPT principles, LTCM’s strategies did not account for “Black Swan” events or extreme market moves. Over-reliance on quantification and undervaluing of qualitative factors, like geopolitical risks, led to its downfall.
5. Growth vs. Value Dichotomy (2010s):
- What Happened: Throughout much of the 2010s, growth stocks (particularly in technology) significantly outperformed value stocks, contrary to the historical premium associated with value investing.
- MPT Shortcoming: MPT posits that higher risks are associated with higher expected returns. However, many growth stocks offered both higher returns and lower volatility than their value counterparts during this period, challenging traditional risk-return dynamics postulated by MPT.
Each of these events underscores the importance of understanding the assumptions and limitations of MPT.
In summary, while MPT offers a foundational framework for understanding risk and return in portfolios, evolving market dynamics, and continued academic inquiry suggest that it should be applied with caution and complemented with other financial tools and insights.
Sources:
- Shiller, R. J. (1992). Market Volatility and Investor Behavior. American Economic Review, 82(2), 58-62.
- Kahneman, D., & Tversky, A. (1979). Prospect Theory: An Analysis of Decision under Risk. Econometrica, 47(2), 263-292.
- Harvey, C. R. (1995). Predictable Risk and Returns in Emerging Markets. Review of Financial Studies, 8(3), 773-816.
- Ibbotson, R. G., & Kaplan, P. D. (2000). Does Asset Allocation Policy Explain 40, 90, or 100 Percent of Performance? Financial Analysts Journal, 56(1), 26-33.
- Radelet, S., & Sachs, J. (1998). The East Asian Financial Crisis: Diagnosis, Remedies, Prospects. Brookings Papers on Economic Activity, 1998(1), 1-90.
- Longin, F., & Solnik, B. (2001). Extreme Correlation of International Equity Markets. The Journal of Finance, 56(2), 649-676.
- Lowenstein, R. (2000). When Genius Failed: The Rise and Fall of Long-Term Capital Management. Random House.
- Daniel, K., & Titman, S. (1997). Evidence on the Characteristics of Cross-Sectional Variation in Stock Returns. The Journal of Finance, 52(1), 1-33.
- Ralfe, J., Speed, C., & Allinson, D. (2004). The Pension Crisis. Lancet, 363(9419), 1343.
- Ang, A., Papanikolaou, D., & Westerfield, M. M. (2014). Portfolio Choice with Illiquid Assets. Management Science, 60(11), 2737-2761.